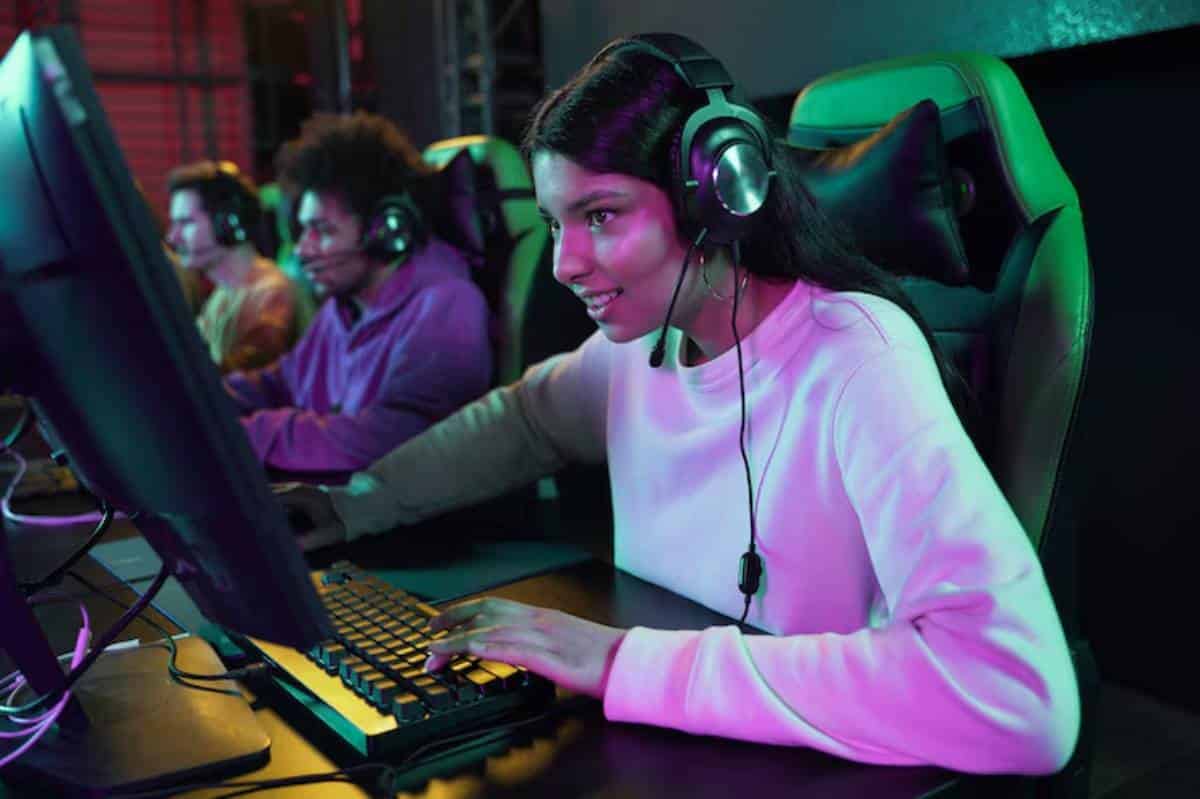
How Colleges Are Using Esports to Boost Enrollment
In the high-stakes world of esports, milliseconds matter. Matches can turn on a single misstep, a poorly timed push, or a lapse in map awareness. For professional players and coaches, staying ahead of the competition is essential. That’s where predictive analytics in esports is beginning to shift the game entirely.
Esports teams, just like traditional sports teams, are now using advanced data tools. They do this to get a competitive edge. These technologies aren’t just for post-match analysis or fan stats anymore. They are now key to real-time gameplay analysis. Teams can use them to predict their opponents’ next moves before they happen.
This article looks at how predictive analytics is changing esports training, match strategy, and live performance. We will explore tools like machine learning models, heat maps, and opponent profiling. These techniques are making foresight a science in digital spaces.
What Is Predictive Analytics in Esports?
Predictive analytics mainly uses past and current data to predict future results. In esports, this involves looking at a lot of gameplay data. This includes player movements, ability usage, kill/death ratios, and economic choices. By finding patterns, we can predict what an opponent might do next.
Key Capabilities:
- Opponent behaviour modelling
- Strategy simulation and scenario testing
- Live match trend tracking
- Automated alerts for playstyle shifts or anomalies
Data-driven foresight can help you proactively counter a strategy instead of just reacting to it.
Why Predictive Analytics Matters in Esports
1. Competitive Margins Are Incredibly Tight
Top-level teams often operate at similar skill levels. Smarter preparation and faster adaptation give you the edge. Esports predictive analytics can help with this.
2. Esports Data Is Abundant and Structured
Unlike many traditional sports, esports produces clean, precise, and instantly accessible data. Every action is logged and time-stamped, making it ideal for analysis.
3. Training Efficiency
AI and predictive modelling help teams run many simulations. This targets practice sessions on specific weaknesses or key plays.
4. Scouting and Counter-Strategising
Coaches can analyse opponents’ habits and adjust playbooks on the spot. This leads to smarter and quicker decisions during matches.
Tools and Technologies Powering Esports Predictive Analytics
Machine Learning Models
AI uses supervised and unsupervised learning to learn player patterns. It can predict decision paths and suggest the best counter-strategies based on past outcomes.
Real-Time Dashboards
Dynamic interfaces share real-time match data. This includes enemy economy in CS:GO, cooldown tracking in League of Legends, and positioning in VALORANT. They give analysts and coaches valuable live insights.
Heat Maps and Movement Analysis
Visual tools show player paths, blind spots, and favoured combat zones. They help teams improve map control and take advantage of predictable patterns.
API Integrations with Game Clients
Modern games now often support API access. This lets developers create custom dashboards to track and analyse gameplay in detail.
Natural Language Processing (NLP)
Some systems now analyse team communications and in-game chat logs. They detect psychological trends or communication issues, which are crucial in long series play.
Real-Time Gameplay Analysis: A Tactical Shift
Real-time gameplay analysis is where predictive analytics proves most potent. Rather than relying solely on post-match reviews, analysts can now adapt strategies mid-match based on live data feeds.
Example Use Cases:
- League of Legends: An analytics engine notes an enemy ADC’s tendency to overextend post-10 minutes—coaches signal a gank opportunity.
- Overwatch 2: A heat map reveals repeated flanking from a Tracer on defence. The team shifts formation to trap the pattern.
- CS:GO: Economic data forecasts a likely force-buy from the opposition, prompting a more conservative push.
These insights don’t aim to take away intuition. Instead, they focus on boosting it with better information.
Predictive Analytics in Training and Preparation
Opponent Profiling
Teams use esports data tools to create predictive models. They examine the opponent’s history. This includes preferred champions, economic choices, first blood habits, rotation timings, and more.
Strategy Simulation
Advanced systems help teams simulate different game states. They can predict how opponents will react to plays like split pushes or double-fake rushes.
Replay Tagging and Auto-Review
AI can now tag key moments in scrims or match footage. This includes misplays, clutches, and rotations. This helps make review sessions more focused and efficient.
Fatigue and Decision Degradation Tracking
Predictive models can spot when a player might underperform. They consider factors like fatigue trends, reaction times, and communication issues. This information is useful for scheduling and managing player loads.
Leading Organisations Leveraging Predictive Analytics
Many leading esports teams and platforms are now using predictive models in their training and match-day routines.
- Team Liquid: Known for using AI-powered scrim reviews and live-match dashboards.
- G2 Esports: Invests in performance analytics that combine gameplay data with biometric tracking.
- Bayes Esports: Provides data analytics solutions for tournament organisers and betting markets, increasingly offering predictive insights to teams as well.
Universities and esports research centres are working with data scientists. They aim to improve prediction engines for competitive and academic use.
Challenges in Applying Predictive Analytics to Esports
While the potential is immense, there are notable challenges:
1. Data Overload
Teams must avoid “analysis paralysis”—where too much data hinders decision-making. The skill lies in filtering noise from insight.
2. Model Bias
AI models are only as good as the data they’re trained on. Changes in meta, patches, or player roles can rapidly invalidate predictions.
3. Human Factors
Predictive models can’t capture everything. They miss emotional state, mental resilience, and player synergy. All these factors influence outcomes.
4. Privacy and Data Ethics
The increasing use of biometric data and player tracking raises concerns around consent, fairness, and information security.
Many teams are combining human insight with AI support. They know that prediction is a tool, not a crutch.
The Future of Esports Data and Prediction
As esports continues to professionalise, predictive analytics will play a more central role in both strategy and performance development.
Emerging Trends:
- Hyper-personalised training based on individual player analytics
- Real-time fan engagement tools that predict in-match outcomes live
- AI-driven coaching assistants capable of offering tactical suggestions during live matches (within rule constraints)
- Cross-game data analysis to scout multi-title athletes and adapt training for genre shifts
The goal isn’t to replace human coaches or instincts—but to enhance them, enabling faster reactions, sharper reads, and smarter strategy.
From Instinct to Insight
In the fast-changing world of competitive gaming, data is now a new currency. Prediction is its most valuable use. Esports predictive analytics is now essential. It helps counter a push before it starts and spot a win condition minutes ahead of the opposing team. It’s becoming a necessity.
As real-time gameplay analysis matures, and esports data tools become more accessible, the line between human intuition and machine insight will continue to blur. Those who embrace this evolution will be the ones rewriting the future of esports.
Act now: If you’re a coach, player, or analyst, check out predictive tools made for your game. Because in a game of milliseconds, foresight is everything.